Experience in Blockchain
Employees
Projects
Blockchain Experts
The digital-first generation of technology has made the need for readily available information a must since a lot can happen over proper datasets. The inevitable growth of artificial intelligence (AI) technology stands as a testament to the statement since all AI solutions use data of some form or another. With recording, storing, and processing real-world data becoming increasingly complex in a world where privacy is increasingly becoming important, synthetic data generation becomes crucial. This type of data is essential for creating high-quality products that can contribute to the betterment of the Web3 industry. This guide provides extensive information on what synthetic data is and how useful it is for businesses in the current era.
Table of Contents
Synthetic Data: An Introduction
Why is Synthesized Data Even Necessary?
Types and Formats of Synthetic Data
Elaborating the Process Behind Synthetic Data Generation
Wide-ranging Use Cases of Synthetic Data
ML Model Training
ML Model Testing
Augmentation
Anonymization
Simulation
Marketing
Cybersecurity
Fraud Detection
Differentiating Synthetic Data from Real and Dummy Counterparts
Ways to Ensure the Quality of Synthetic Data
Popular Synthetic Data Companies in the Current Age
What Does the Future Look Like?
Conclusion
Synthetic Data: An Introduction
- As we saw in the introduction, synthetic data’s importance has skyrocketed. But what is synthetic data, after all? Synthetic data is generated by AI-based solutions based on information they were fed. In this case, the resulting information closely resembles the original data in terms of statistical properties and characteristics, although no sensitive data is revealed.
- Synthetic data is utilized for various purposes, especially in the artificial intelligence industry, where data privacy has become a concerning point. In this regard, the unsavory relationship between the public and giant tech corporations has elevated the need for artificially synthesized data that safeguards user privacy from the core.
Why is Synthesized Data Even Necessary?
If you are wondering why synthetic data generation is even needed in an era when information of all kinds is readily available from the Internet, think again. Despite data becoming available to anyone, not everyone wants their personal information used by a random AI solution for a random use case without their permission.
The following points shed light on the necessity for using synthetic data:
-
Data Privacy
Using synthetic data preserves the privacy of sensitive information at a time when leakage of personal information has become a common occurrence. While resembling personal information, synthetic data only resembles and does not represent true data, easing concerns about user privacy.
Data Diversity
Tapping into artificially generated information from AI algorithms diversifies the available data, especially when the datasets are small in volume. Most times, acquiring information from the real world incurs a lot of time, effort, and money, which could be simply prohibitive for most projects, and synthetic data can step up to the occasion brilliantly.
Economical
Utilizing synthetic data for any purpose (academic/research/commercial) incur lesser costs than working with real-world information that could come with a longer workflow to make the data workable for the algorithms. Since AI is used for generating data, resource requirements decrease, making it easier for small-scale ventures to work efficiently despite resource constraints.
Scalability
With synthetic datasets, scalability becomes a real possibility since large volumes of information can be generated on demand, an impossible task when it comes to real-world data. The quality of data, though, depends on the AI-based algorithms used and the quality of information fed into the data generation model.
Model Testing
Synthetic data can come as a great relief for machine learning models by allowing them to test their data integrity and performance without risking the personal information of any real-world entities. With thousands of models under development, synthetic data is a real boon to startups and enterprises alike, who previously had limited access to information.
Types and Formats of Synthetic Data
Like any other data, synthetic data comes in all types and formats, easing the process for users using AI technology for various industrial and research use cases. The below subsections discuss the types and formats of synthetic data in detail.
Types of Synthetic Data
1. Fully Synthetic Data
Fully synthetic data is fully generated by AI algorithms and does not contain any original information. This kind of data is useful for applications where using original data might endanger the well-being of individuals or organizations. Multiple imputations and bootstrap methods are popular ways to create fully synthetic data.
2. Partially Synthetic Data
Partially synthetic data could contain bits of original information, and the AI algorithm would replace selected aspects of the data with random information. This is useful in applications where only some points of personal information are deemed too sensitive to reveal than others. Model-based techniques and multiple imputations are popular ways to form partially synthetic data.
3. Hybrid Synthetic Data
Hybrid synthetic data contains a mix of real and artificial data, where synthetic data is used in place of random aspects of real-world data. Due to the level of complexity involved, this type of data proves to be extremely useful despite incurring higher memory space and processing time.
Formats of Synthetic Data
1. Textual
Textual synthetic data is generated by AI algorithms that resemble realistic text. Although replicating the tone of realistic content was initially challenging, the advent of advanced machine learning models and natural language processing algorithms make it possible step by step.
2. Tabular
Tabular synthetic data closely resembles information in tabulated forms that can be used for classification and comparison. The increasing importance of data analysis and research has made the requirements clear for generated data in a tabular form that can help accelerate advancements in various fields.
3. Multimedia
Multimedia synthetic data usually involves images, audio, and videos created using AI models. These can be utilized to train models using computer vision to find and comprehend data in digital format. As the need for digital identification increases, this kind of synthetic data can boost developments.
Elaborating the Process Behind Synthetic Data Generation
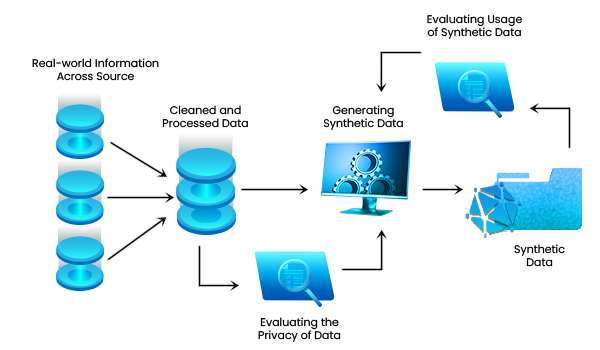
The process behind synthetic data generation involves multiple steps that require careful attention and execution to get results of the desired quality. Forming artificial data resembling real-world information for various industrial applications demands hectic efforts from people involved in setting up the AI model. The steps below explain how synthetic data is generated from scratch.
1. Collect Real-world Information
The process begins with collecting real-world information necessary for the synthetic model from various sources. Data can be collected from databases, APIs, data providers, and other sources relevant to the required domain. The information should have a wide scope inside the target field and represent various scenarios and examples without compromises.
2. Clean and Harmonize Data
The data should be harmonized and cleaned after collecting information from real-world sources related to the target domain. Firstly, data should be processed based on various filtering points, after which any information that is redundant, duplicated, and missing should be cleaned to provide clear datasets that can be used to create synthetic data.
3. Evaluate Privacy of Data
The freshly prepared data should be evaluated for privacy implications based on the domain and the regions where it will be used. Alongside this, data will also be scoured for any information that could be categorized as sensitive personal data, which should be handled properly according to global and regional regulations for digital data handling ans usage.
4. Generate Synthetic Data
After preparing the necessary datasets, the model or algorithm for generating synthetic data is built. It should be created to create data that resembles original information in terms of statistical properties and recognizable patterns, which can open up research opportunities in many fields where data is scarce or prohibitively expensive.
Develop an Exclusive Model for Creating Artificial Information with Our Synthetic Data Company!
Wide-ranging Use Cases of Synthetic Data
Synthetic data has various use cases in a time when information is wealth, with various industries finding the need for more data imperative. While having real-world information always helps, the difficulties associated with acquiring it make it challenging for startup projects and researchers. Synthetic data generation, in this case, becomes helpful since it resembles original data forms. The sections below discuss some of the cases of synthetic data being used in the modern day.
-
ML Model Training
Synthetic data can be used to train machine learning models for use cases where access to real-world information is limited, costly, or sensitive. Using generated datasets replicating real-world information, models can be trained to enhance their generalization capabilities, which could be useful in the long run.
ML Model Testing
Artificially generated data can be used to test and validate the performance of ML-based models under various conditions. Doing so enables developers to conduct extensive testing and quality assurance practices without needing to collect actual data, which would be infeasible for most projects.
Augmentation
Generative information from AI algorithms can be used for data augmentation, a practice where training datasets are diversified and widened. This can come in handy in situations where an ample volume of real-world information is not readily available or hard to acquire to train an AI model.
Anonymization
Synthetic data from AI solutions can be used for anonymization, where alternative information is created in place of sensitive and personal real-world data. Using anonymized information based on synthetic datasets can be crucial for training AI models without worrying about ethical and legal implications surrounding the use of real-world information.
Simulation
Artificial data can be used in simulation and modeling to develop virtual environments and scenarios that could not be formed under real-world conditions. High-quality simulations can be created without compromising the privacy of any individual or organization by tapping into data that only replicates the statistical behavior and patterns noticed in real-world information.
Marketing
Synthetic data generation can be greatly useful for promotional campaigns. It lets marketers gain diverse insights and perspectives that can help them improve their performance. By creating synthetic customer personas, marketing teams can frame a comprehensive campaign without worrying about the implications of handling sensitive data of real people.
Cybersecurity
Data generated using synthetic algorithms can play a pivotal role in the cybersecurity field to let projects strengthen their capabilities to fight harder cyber attacks. Using artificial information here can be helpful for cybersecurity teams since it can offer diversified data and scenarios that could be difficult to construct in the real world.
Fraud Detection
Another use case where synthetic data can be critical is fraud detection in the financial sector. Artificially generated information can be used to recreate situations and patterns that reflect fraudulent behavior in financial transactions without needing to utilize the personal data of others. Doing so can help financial institutions like banks stay up with the improved tactics followed by malicious actors.
Differentiating Synthetic Data from Real and Dummy Counterparts
If you have wondered how synthetic data is different from real and dummy data, you are not alone. While you might have recognized the apparent differences between synthetic and real data, there is a high chance that you might think that synthetic data is the same as dummy data. This section is here to resolve your concerns with the correct differences.
Firstly, let us differentiate synthetic data from real data. Real data is obviously the information one collects from real-world resources, while synthetic data, as we know, is generated using machine learning models or algorithms. As we emphasized earlier, real data does not always represent the true diversity of possibilities, and the chances for acquiring such voluminous information are incredibly challenging for a number of reasons.
It is time to explore how synthetic data and dummy data differ from each other. Dummy data is often created manually by developers involved in testing applications, whereas synthetic data is generated by sophisticated algorithms. The latter typically reflect more diversity than the former due to the intensive training the algorithms undergo, not to mention developers might not always identify all the testing possibilities surrounding an application.
Ways to Ensure the Quality of Synthetic Data
AI model developers should ensure that the quality of synthetic data they use is appropriate for the use case their model will be working on. There are multiple ways to ensure the quality of artificially generated data for training ML-based models, some of which are discussed below:
Using an Apt Generative Model
Using an appropriate generative AI model with the necessary configuration is vital for ensuring the quality of synthetic data. Adjusting the parameters just right could be crucial in enhancing the quality of output from meager to excellent, which takes a lot of exploration and experimentation while abiding by privacy policies.
Validating Against Known Values
To ensure that the resulting synthetic data is of higher quality, validating the output against known values in the real-world data source could be helpful. Analyzing the statistical parameters of both datasets and checking their resemblance is key to determining the quality of generated data.
Testing Regularly for Defects
Regularly testing the synthetic data output for potential defects can pave the way for improving the quality of output. With every testing iteration, newer errors will be found, which would enhance the output’s nature, ultimately establishing the highest quality possible. Getting insights from professionals in the relevant domain could be helpful as well.
Evaluating Various Trade-offs
Evaluating trade-offs in aspects like privacy and utility of generative data could help identify the quality of information. Adequate measures can ensure ample levels of privacy while preserving the usefulness of data without causing many problems that could lead to unsavory situations.
Become a Pioneer in the AI Industry with a High-Class Synthetic Data Generation Model!!
Popular Synthetic Data Companies in the Current Age
Although synthetic data looks like a novel domain, it has existed for a long time, bringing several noticeable players into the industry. Numerous synthetic data companies have come up with their own models for generating artificial data resembling real-world data while not leaking sensitive information. The list below shows some of the top ventures offering synthetic data models for business and enterprise needs:
- Accelario
- BetterData
- Facteus
- Gretel.ai
- MDClone
- MOSTLY AI
- AI Reverie
- Bifrost
- ElevenLabs
- Parallel Domain
- Synthesis AI
While some of these companies focus on structured synthetic data, others focus on unstructured synthetic data. For refreshers, structured synthetic data shows clear relations between data points and is mostly in tabular form. On the other hand, unstructured synthetic data does not have a preset structure (Examples, text, image, video) and needs new methods for analysis.
What Does the Future Look Like?
The future of synthetic data generation looks bright since the space is improving at a quick pace. With various points of concern, improvising the quality of synthetic data output is deemed necessary, and efforts are ongoing to maximize the possibilities. The following points shed light on aspects of the future of the synthetic data domain:
- Scaling synthetic data with equal emphasis on quality and quantity by understanding the dynamics of consistency of the generated information.
- Enhancing the diversity and quality of synthetic data based on specific parameters is a real possibility that could prove beneficial for researchers across industries to improve products and services.
- Ensuring high fidelity of the generated output is another area for improvement with AI models increasing in complexity. This can be useful to simulate challenging situations with multiple variable factors.
- Another field where synthetic data can improve is computer vision for various industries. This technology can help test products under conditions that are impossible to replicate in the real world.
Conclusion
We hope the guide enlightened you on the intricacies behind synthetic data generation. With original data becoming increasingly challenging for most people to obtain, using artificial data to replicate the original data’s parameters could be a blessing. Startups, enterprises, and researchers could tap into the potential of synthetic data to train AI models that can function across situations without needing to access sensitive real-world information for training purposes. If you are looking to make the most of the brewing revolution in the world of digital data and artificial intelligence, now could be the perfect time to start, and getting expert assistance from companies like ours is recommended.